Oversampling and undersampling in data analysis are techniques used to adjust the class distribution of a data set (i.e. the ratio between the different classes/categories represented). These terms are used both in statistical sampling, survey design methodology and in machine learning.
- On Under Over For Example Parts Of Speech
- Over Under Example
- What Does Over Under Mean
- Over Under Examples
Oversampling and undersampling are opposite and roughly equivalent techniques. There are also more complex oversampling techniques, including the creation of artificial data points with algorithms like Synthetic minority oversampling technique.[1][2]
Motivation for oversampling and undersampling[edit]
Both oversampling and undersampling involve introducing a bias to select more samples from one class than from another, to compensate for an imbalance that is either already present in the data, or likely to develop if a purely random sample were taken. Data Imbalance can be of the following types:
- Under-representation of a class in one or more important predictor variables. Suppose, to address the question of gender discrimination, we have survey data on salaries within a particular field, e.g., computer software. It is known women are under-represented considerably in a random sample of software engineers, which would be important when adjusting for other variables such as years employed and current level of seniority. Suppose only 20% of software engineers are women, i.e., males are 4 times as frequent as females. If we were designing a survey to gather data, we would survey 4 times as many females as males, so that in the final sample, both genders will be represented equally. (See also Stratified Sampling.)
- Under-representation of one class in the outcome (dependent) variable. Suppose we want to predict, from a large clinical dataset, which patients are likely to develop a particular disease (e.g., diabetes). Assume, however, that only 10% of patients go on to develop the disease. Suppose we have a large existing dataset. We can then pick 1/9th the number of patients who did not go on to develop the disease for every one patient who did.
Example: The Over/Under for Super Bowl LII was 47.5 points. The Philadelphia Eagles defeated the New England Patriots, 41–33, meaning the teams combined to score 74 points. If you bet the over at. Definition of under1 preposition in Oxford Advanced Learner's Dictionary. Meaning, pronunciation, picture, example sentences, grammar, usage notes, synonyms and more. We use cookies to enhance your experience on our website, including to provide targeted advertising and track usage.
Oversampling is generally employed more frequently than undersampling, especially when the detailed data has yet to be collected by survey, interview or otherwise. Undersampling is employed much less frequently. Overabundance of already collected data became an issue only in the 'Big Data' era, and the reasons to use undersampling are mainly practical and related to resource costs. Specifically, while one needs a suitably large sample size to draw valid statistical conclusions, the data must be cleaned before it can be used. Cleansing typically involves a significant human component, and is typically specific to the dataset and the analytical problem, and therefore takes time and money. For example:
- Domain experts will suggest dataset-specific means of validation involving not only intra-variable checks (permissible values, maximum and minimum possible valid values, etc.), but also inter-variable checks. For example, the individual components of a differential white blood cell count must all add up to 100, because each is a percentage of the total.
- Data that is embedded in narrative text (e.g., interview transcripts) must be manually coded into discrete variables that a statistical or machine-learning package can deal with. The more the data, the more the coding effort. (Sometimes, the coding can be done through software, but somebody must often write a custom, one-off program to do so, and the program's output must be tested for accuracy, in terms of false positive and false negative results.)
For these reasons, one will typically cleanse only as much data as is needed to answer a question with reasonable statistical confidence (see Sample Size), but not more than that.
Oversampling techniques for classification problems[edit]
Random oversampling[edit]
Random Oversampling involves supplementing the training data with multiple copies of some of the minority classes. Oversampling can be done more than once (2x, 3x, 5x, 10x, etc.) This is one of the earliest proposed methods, that is also proven to be robust. [3] Instead of duplicating every sample in the minority class, some of them may be randomly chosen with replacement.
SMOTE[edit]
There are a number of methods available to oversample a dataset used in a typical classification problem (using a classification algorithm to classify a set of images, given a labelled training set of images). The most common technique is known as SMOTE: Synthetic Minority Over-sampling Technique.[4] To illustrate how this technique works consider some training data which has s samples, and f features in the feature space of the data. Note that these features, for simplicity, are continuous. As an example, consider a dataset of birds for classification. The feature space for the minority class for which we want to oversample could be beak length, wingspan, and weight (all continuous). To then oversample, take a sample from the dataset, and consider its k nearest neighbors (in feature space). To create a synthetic data point, take the vector between one of those k neighbors, and the current data point. Multiply this vector by a random number x which lies between 0, and 1. Add this to the current data point to create the new, synthetic data point.
Many modifications and extensions have been made to the SMOTE method ever since its proposal. [5]
ADASYN[edit]
The adaptive synthetic sampling approach, or ADASYN algorithm,[6] builds on the methodology of SMOTE, by shifting the importance of the classification boundary to those minority classes which are difficult. ADASYN uses a weighted distribution for different minority class examples according to their level of difficulty in learning, where more synthetic data is generated for minority class examples that are harder to learn.
Undersampling techniques for classification problems[edit]
Random undersampling[edit]
Randomly remove samples from the majority class, with or without replacement. This is one of the earliest techniques used to alleviate imbalance in the dataset, however, it may increase the variance of the classifier and may potentially discard useful or important samples. [5]
Cluster[edit]
Cluster centroids is a method that replaces cluster of samples by the cluster centroid of a K-means algorithm, where the number of clusters is set by the level of undersampling.
Tomek links[edit]
Tomek links remove unwanted overlap between classes where majority class links are removed until all minimally distanced nearest neighbor pairs are of the same class. A Tomek link is defined as follows: given an instance pair , where and is the distance between and , then the pair is called a Tomek link if there's no instance such that or . In this way, if two instances form a Tomek link then either one of these instances is noise or both are near a border. Thus, one can use Tomek links to clean up overlap between classes. By removing overlapping examples, one can establish well-defined clusters in the training set and lead to improved classification performance.
Undersampling with ensemble learning
A recent study shows that the combination of Undersampling with ensemble learning can achieve better results, see IFME: information filtering by multiple examples with under-sampling in a digital library environment.[7]
Additional techniques[edit]
It's possible to combine oversampling and undersampling techniques into a hybrid strategy. Common examples include SMOTE and Tomek links or SMOTE and Edited Nearest Neighbors (ENN). Additional ways of learning on imbalanced datasets include weighing training instances, introducing different misclassification costs for positive and negative examples and bootstrapping.[8]
Implementations[edit]
- A variety of data re-sampling techniques are implemented in the imbalanced-learn package [1] compatible with Python's scikit-learn interface. The re-sampling techniques are implemented in four different categories: undersampling the majority class, oversampling the minority class, combining over and under sampling, and ensembling sampling.
- The Python implementation of 85 minority oversampling techniques with model selection functions are available in the smote-variants [2] package.
See also[edit]
References[edit]
- ^ abhttps://github.com/scikit-learn-contrib/imbalanced-learn
- ^ abhttps://github.com/analyticalmindsltd/smote_variants
- ^Ling, Charles X., and Chenghui Li. 'Data mining for direct marketing: Problems and solutions.' Kdd. Vol. 98. 1998.
- ^https://www.cs.cmu.edu/afs/cs/project/jair/pub/volume16/chawla02a-html/chawla2002.html
- ^ abChawla, Nitesh V.; Herrera, Francisco; Garcia, Salvador; Fernandez, Alberto (2018-04-20). 'SMOTE for Learning from Imbalanced Data: Progress and Challenges, Marking the 15-year Anniversary'. Journal of Artificial Intelligence Research. 61: 863–905. doi:10.1613/jair.1.11192. ISSN1076-9757.
- ^http://sci2s.ugr.es/keel/pdf/algorithm/congreso/2008-He-ieee.pdf
- ^Zhu, Mingzhu; Xu, Chao; Wu, Yi-Fang Brook (2013-07-22). IFME: information filtering by multiple examples with under-sampling in a digital library environment. ACM. pp. 107–110. doi:10.1145/2467696.2467736. ISBN9781450320771.
- ^Haibo He; Garcia, E.A. (2009). 'Learning from Imbalanced Data'. IEEE Transactions on Knowledge and Data Engineering. 21 (9): 1263–1284. doi:10.1109/TKDE.2008.239.
- Chawla, Nitesh V. (2010) Data Mining for Imbalanced Datasets: An Overviewdoi:10.1007/978-0-387-09823-4_45 In: Maimon, Oded; Rokach, Lior (Eds) Data Mining and Knowledge Discovery Handbook, Springer ISBN978-0-387-09823-4 (pages 875–886)
- Lemaître, G. Nogueira, F. Aridas, Ch.K. (2017) Imbalanced-learn: A Python Toolbox to Tackle the Curse of Imbalanced Datasets in Machine Learning, Journal of Machine Learning Research, vol. 18, no. 17, 2017, pp. 1-5.
As well as the familiar equals sign (=) it is also very useful to show if something is not equal to (≠) greater than (>) or less than (<)
These are the important signs to know:
= | When two values are equal | example: 2+2 = 4 |
≠ | When two values are definitely not equal | example: 2+2 ≠ 9 |
< | When one value is smaller than another | example: 3 < 5 |
> | When one value is bigger than another | example: 9 > 6 |
Less Than and Greater Than
The 'less than' sign and the 'greater than' sign look like a 'V' on its side, don't they?
To remember which way around the '<' and '>' signs go, just remember:
- BIG > small
- small < BIG
Greater Than Symbol: BIG > small
Example:
10 > 5
'10 is greater than 5'
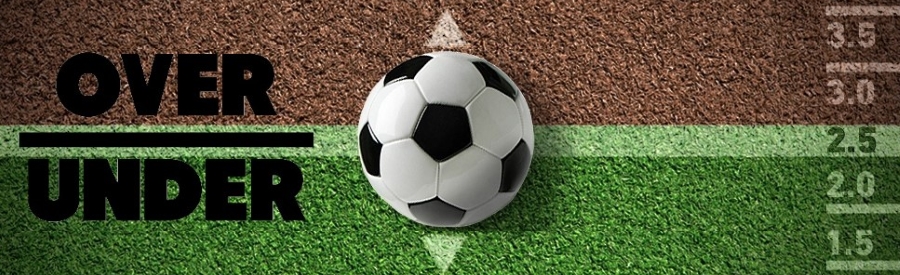
Or the other way around:
5 < 10
'5 is less than 10'
Do you see how the symbol 'points at' the smaller value?
... Or Equal To ...
Sometimes we know a value is smaller, but may also be equal to!
Example, a jug can hold up to 4 cups of water.
So how much water is in it?
It could be 4 cups or it could be less than 4 cups: So until we measure it, all we can say is 'less than or equal to' 4 cups.
To show this, we add an extra line at the bottom of the 'less than' or 'greater than' symbol like this:
The 'less than or equal to' sign: | ≤ |
The 'greater than or equal to' sign: | ≥ |
All The Symbols
Here is a summary of all the symbols:
Words | |
---|---|
= | 1 + 1 = 2 |
not equal to | |
> | 5 > 2 |
less than | |
≥ | marbles ≥ 1 |
less than or equal to |
Why Use Them?
Because there are things we do not know exactly ...
So we have ways of saying what we do know (which may be useful!)
Example: John had 10 marbles, but lost some. How many has he now?
Answer: He must have less than 10:
Marbles < 10
If John still has some marbles we can also say he has greater than zero marbles:
Marbles > 0
But if we thought John could have lost all his marbles we would say
Marbles ≥ 0
In other words, the number of marbles is greater than or equal to zero.
Combining
We can sometimes say two (or more) things on the one line:
Example: Becky starts with $10, buys something and says 'I got change, too'. How much did she spend?
Answer: Something greater than $0 and less than $10 (but NOT $0 or $10):
'What Becky Spends' > $0
'What Becky Spends' < $10
This can be written down in just one line:
$0 < 'What Becky Spends' < $10
That says that $0 is less than 'What Becky Spends' (in other words 'What Becky Spends' is greater than $0) and what Becky Spends is also less than $10.
Notice that '>' was flipped over to '<' when we put it before what Becky spends. Always make sure the small end points to the small value.
Changing Sides
We saw in that previous example that when we change sides we flipped the symbol as well.
This: | is the same as this: | Just make sure the small end points to the small value! Here is another example using '≥' and '≤': Example: Becky has $10 and she is going shopping. How much will she spend (without using credit)?Answer: Something greater than, or possibly equal to, $0 and less than, or possibly equal to, $10: Becky Spends ≥ $0 This can be written down in just one line: $0 ≤ Becky Spends ≤ $10 A Long Example: Cutting RopeHere is an interesting example I thought of: Example: Sam cuts a 10m rope into two. How long is the longer piece? How long is the shorter piece?Answer: Let us call the longer length of rope 'L', and the shorter length 'S' L must be greater than 0m (otherwise it isn't a piece of rope), and also less than 10m: L > 0 So: 0 < L < 10 ![]() That says that L (the Longer length of rope) is between 0 and 10 (but not 0 or 10) The same thing can be said about the shorter length 'S': 0 < S < 10 But I did say there was a 'shorter' and 'longer' length, so we also know: S < L (Do you see how neat mathematics is? Instead of saying 'the shorter length is less than the longer length', we can just write 'S < L') We can combine all of that like this: 0 < S < L < 10 That says a lot: 0 is less that the short length, the short length is less than the long length, the long length is less than 10. On Under Over For Example Parts Of SpeechReading 'backwards' we can also see: 10 is greater than the long length, the long length is greater than the short length, the short length is greater than 0. It also lets us see that 'S' is less than 10 (by 'jumping over' the 'L'), and even that 0<10 (which we know anyway), all in one statement. NOW, I have one more trick. If Sam tried really hard he might be able to cut the rope EXACTLY in half, so each half is 5m, but we know he didn't because we said there was a 'shorter' and 'longer' length, so we also know: Over Under ExampleS<5 and L>5 We can put that into our very neat statement here: 0 < S < 5 < L < 10 And IF we thought the two lengths MIGHT be exactly 5 we could change that to 0 < S ≤ 5 ≤ L < 10 An Example Using AlgebraOK, this example may be complicated if you don't know Algebra, but I thought you might like to see it anyway: What Does Over Under MeanExample: What is x+3, when we know that x is greater than 11?If x > 11 , then x+3 > 14 Over Under Examples(Imagine that 'x' is the number of people at your party. If there are more than 11 people at your party, and 3 more arrive, then there must be more than 14 people at your party now.) |